Here’s a fun challenge: try going a day without hearing or reading something about Artificial Intelligence (AI). Trust me, I’ve tried. I thought I was doing well until my washing machine’s “AI Suggested” cycle settings appeared on its little screen and I was sucked back into the vortex of existential concern for humanity. More than just a buzzword tossed around by tech enthusiasts and futurists, AI has leapt out of the big tech keynote presentations to become a recurring topic in our mainstream conversations.
As AI continues its evolution, we find ourselves standing at a critical crossroads. The decisions we make now about how to guide and shape this groundbreaking technology will not only determine the future of AI but also, potentially, our own.
Here’s the kicker though: there’s a real danger that excessive human interference and over-regulation could choke AI’s ‘natural progression’ (and yes, I’m aware of the irony of using the term ‘natural’ here). I am sure we can all agree that it is natural for us as humans to be wary of AI’s capabilities. Our fears are deeply ingrained in our instinctual drive for self-preservation and personal gain, but it’s essential for us to take a step back. We must reflect on the impact we have on this technology, the responsibility we bear, and the consequences of our actions, as trying to stop AI is akin to stopping humanity’s next great evolutionary step.
Now, let’s get one thing clear. I’m not suggesting we should hand over the keys to the kingdom to our AI overlords just yet. However, there will come a time where we must be willing to give AI technologies a degree of autonomy – if we have done a good job of training it, it should be able to act in our interests, and that of the world we live in. But let’s pause for a second before we dive deeper into this discussion, and let’s get some foundational topics out of the way, such as what AI is and how it works (for, or against us).
What is Artificial Intelligence?
As the name implies, it’s all about creating smart machines that can act like humans. This means they can learn, reason, solve problems, make decisions, and even get creative in areas like image and music creation. It’s like having a digital brain that can mimic human thinking.
How does AI accomplish this? The secret lies in complex algorithms and computational models. These allow AI to analyze gargantuan amounts of data, spot patterns, generate insights, or make predictions. And while this might sound like the plot of an erotic novel for data engineers, training an AI model isn’t as complex (at least in theory) as you might think.
There are many ways to train an AI model, but three methods are used most commonly. Let’s break them down with some real-world examples:
Supervised Learning
Supervised Learning is like an AI classroom where the AI is the eager student, and the human is the attentive teacher. The goal is to train a model to map input data (the lesson) to the correct output labels (the right answer). But what does this look like in reality?
Imagine you have a very handsome friend, let’s call him James, who has developed a sudden passion for tea. Not just one type of tea, mind you, but the vast array of exotic teas from around the globe. To aid in his noble endeavor, you provide James with a dataset of different teas, each labelled with its distinct characteristics. By studying (and sipping) these examples, he learns to match specific features with each type of tea.
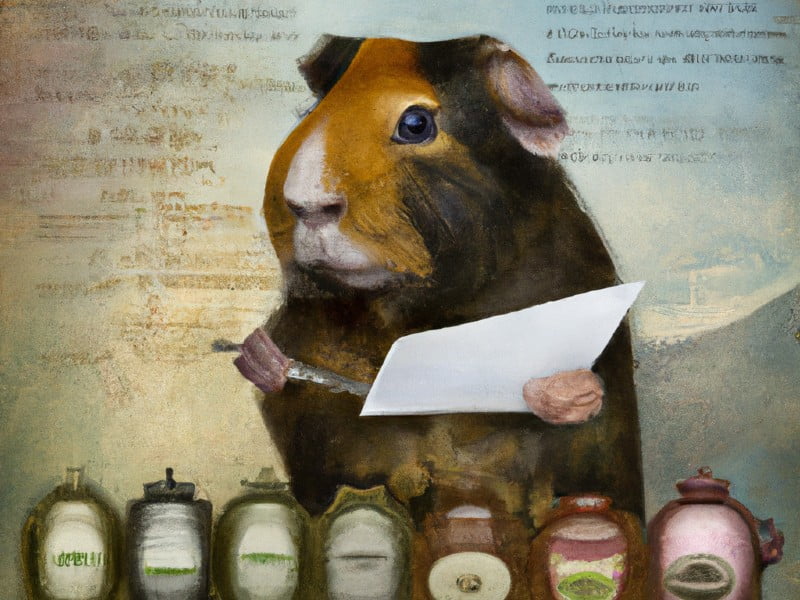
AI models learn in a similar way. Like the best teachers, we guide them through the learning process, providing them with labeled datasets to learn from. When the AI makes predictions, we test them and provide tweaks and corrections to the training, just like any good teacher would. Sure, the AI might make mistakes, but that’s part of the learning process.
Unsupervised Learning
Unsupervised Learning is a bit like being a detective, searching for patterns and relationships in unlabeled data. The idea is to let the learning process loose on the data without any predefined directions or instructions. It’s like throwing Watson into a room full of clues and watching him work his magic.
Imagine you’re stuck on a rainy day in Tasmania with nothing but a bag full of different kinds of apples. Just like our dear Watson, you have no clue about the varieties of these apples. So, what do you do? You start examining them, looking at their size, color, texture, and even taking a bite to check their taste. As you dig in, you start noticing patterns and similarities. You group similar apples together and voila! You’ve just discovered the world of unsupervised learning.
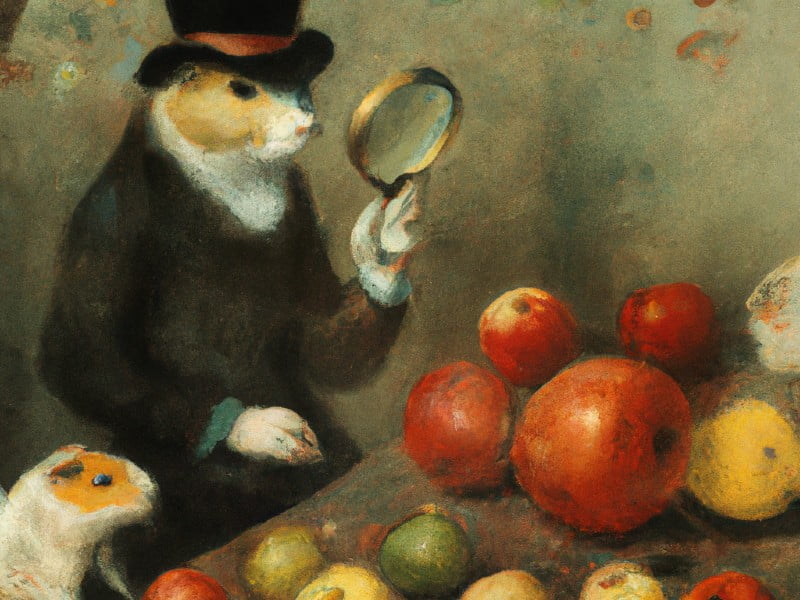
This Sherlock Holmes-style AI sleuthing has a range of applications. It’s used in customer segmentation, recommendation systems, image and text analysis, anomaly detection, and more. Unsupervised learning can unearth hidden treasures in data that even the keenest human eye might miss.
Reinforcement Learning
Picture this: You wake up one day with an insatiable urge to learn the bagpipes (let’s be real, we’ve all had those mornings). You have zero experience with this rather grand instrument, so how do you begin? You start experimenting with fingerings, breath control, and bag pressure techniques.
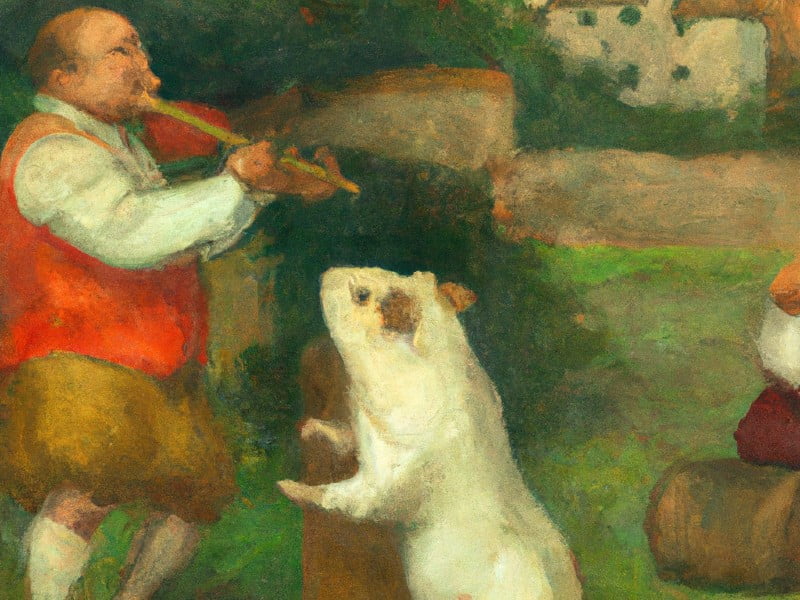
As you’re playing (or trying to), you get feedback from your environment. For example, your neighbors might lob the apples they’re sorting at your window if your bagpipe rendition of ‘Oops I Did it Again’ by Britney Spears is less than optimal. Each time you receive feedback, you adjust your playing technique to produce a more melodious sound and avoid future apple attacks.
This is reinforcement learning in a nutshell: an agent learns to make sequential decisions by interacting with an environment to maximize rewards and minimize penalties. It’s inspired by how we humans and animals learn from our actions.
Reinforcement learning is like a supercharged bagpipe lesson, but it has applications far beyond irritating your neighbors. It’s been used in everything from robotics control and autonomous driving to game playing (such as AlphaGo), recommendation systems, and resource management. The goal is to create AI agents capable of learning optimal behaviors, even in complex and uncertain environments.
Flavors of Artificial Intelligence
So, at this point we have covered how AI learns, but that’s just the start. The type of learning method we pick for an AI is often based on its intended use. With that, let’s dive into the juicy stuff and cover the different types of AI.
Generative AI
Generative AI has made some waves in recent times, and I am sure you have heard of it without even realizing it. It’s been transforming numerous industries, and it’s made some of us question what it means to be human. After all, creativity has long been viewed as a uniquely human trait, and now we’re seeing machines produce work that could pass as human-made.
Take OpenAI’s GPT, which stands for Generative Pre-trained Transformer (in case you need it for pub trivia or as a pick-up line). It uses deep learning and neural networks to generate text that’s coherent and contextually relevant. It’s been trained on a massive amount of data, from books to articles to websites. The result? Text that’s so human-like it’s often hard to tell it was written by a machine.
But generative AI isn’t just about creating text. Microsoft has incorporated generative AI technology into search and browser, using GPT to redefine how we interact with the web as a whole, making the process more intuitive for humans with a conversational interface.
Outside of the world of text generation, platforms like Midjourney and DALL·E 2 have been showing great promise in the generation of photorealistic images, even going so far as recreating works of art by known artists. By using generative AI techniques to understand and mimic artistic styles, users can create unique, high-quality images. While it’s a huge win for industries like graphic design, advertising, and entertainment, the blade cuts both ways, with many concerns about how this tech might affect their jobs.
But generative AI isn’t just limited to text and images. It’s also making advancements in music generation. By analyzing patterns and structures in existing music, generative AI models can create original pieces. It can assist musicians in the creative process, with technologies like IBM’s Watson Beat inspiring new musical ideas, and even generating royalty-free music for use by content creators across a broad array of platforms, lowering the barrier to entry for high-quality production of content.
Natural Language Processing
Let’s dive a level deeper, and get into the comprehension of human writing. Imagine being at a tea party with AI as your co-host, churning out jokes, interpreting your guest’s mood from their chats, and even translating their foreign phrases into English. Sounds like a wild tea party, right? Welcome to the world of Natural Language Processing, or NLP.
NLP is a blend of linguistics, computer science, and machine learning, which gives AI the ability to understand, interpret, and communicate in human language (even Tasmanian!). It’s like a universal translator from a sci-fi franchise, but instead of alien tongues, it deciphers our tweets, emails, and everything in between. And it’s not just about understanding; NLP is also an excellent tool for extracting valuable insights from textual data.
One of the most interesting uses of NLP (in my opinion) is sentiment analysis. Picture a mood ring, but instead of changing colors, it sifts through your tweets, reviews, or customer feedback, identifying keywords, linguistic patterns, and context to gauge the sentiment. It tells you whether the people writing about your organization are happy, upset, indifferent, or just need a cup of tea, some relaxing bagpipe music, and a delicious crunchy apple).
Sentiment analysis is like a cheat sheet for brand monitoring, market research, customer feedback analysis, and social media monitoring. It gives businesses a sneak peek into public opinion, helps evaluate customer satisfaction, and supports data-driven decisions based on sentiment trends.
Another area where NLP shines is language translation. Thanks to NLP, machine translation systems like Google Translate and DeepL Translator have become much more than just phrasebooks. They can now analyze grammatical structures, semantics, and contextual cues within a sentence to churn out translations that are more accurate and coherent (a lifesaver for me as I learn Spanish).
Computer Vision
Ever wondered if computers could see the world just like us? Enter the realm of computer vision, an exciting subset of artificial intelligence and computer science that’s all about enabling machines to comprehend the visual world around them (a particular topic of interest for me).
Let’s start with a topic we have all heard of (for better or worse) thanks to Elon Musk: self-driving vehicles. These autonomous marvels use computer vision algorithms to take in their surroundings and make decisions in real-time. They’re like your car’s eyes, using cameras, sensors, and advanced image processing techniques to recognize objects like pedestrians, traffic signs, and other vehicles. By analyzing visual data and pulling out relevant features, they can help your car navigate safely and make intelligent driving decisions (because let’s be honest, some drivers need to scrape together all the intelligence they can get).
Next, to the world of medical imaging, where computer vision plays a critical role. Algorithms analyze images from X-rays, MRIs, and CT scans to spot abnormalities, tumors, and other medical conditions. They’re like high-tech medical detectives, automatically identifying patterns and anomalies in images. This not only makes it easier for healthcare professionals to diagnose and treat diseases, but also improves patient care by enabling faster and more precise analysis of medical data.
Over in the retail industry, computer vision is changing the game. Retailers use it to understand customer behavior, fine-tune store layouts, and enhance the overall shopping experience. It’s like having a super-smart store assistant who can track customer movements, process demographics, and understand customer preferences (though some notable companies in Australia are having to face the music for doing this without consent). This data helps retailers personalize marketing campaigns, optimize inventory management, and provide targeted recommendations to customers. Plus, with automated stock tracking, shelf monitoring, and efficient product replenishment, computer vision is also a big help when it comes to inventory management.
And let’s not forget security systems (one of my favorite use cases, being a true crime fan). Video surveillance systems use computer vision algorithms to monitor live feeds, spot suspicious activities, and recognize faces or license plates. It’s like having a digital security guard that never sleeps, always ready to identify potential security threats, alert security personnel, and provide evidence for forensic investigations. Thanks to computer vision, biometric identification systems can recognize individuals based on their facial features or other unique characteristics (though, this technology leaves a lot to be desired, and despite this, police in my home country of Australia are still using it as an investigative tool).
Automatic Speech Recognition
Imagine a world where machines could understand our spoken words and respond accurately – nifty, right? Well, you don’t need to imagine anymore (although, those of us with Google Assistant-enabled devices might feel differently, but don’t get me started). Thanks to speech recognition technology, or Automatic Speech Recognition (ASR), machines are getting quite good at understanding and processing our speech.
Perhaps the most familiar application of speech recognition is in voice assistants. From Amazon’s Alexa to Apple’s Siri, Google Assistant, and Microsoft’s Cortana (can’t forget Cortana, although Microsoft seems to have) – these handy virtual assistants use speech recognition algorithms to understand and respond to your commands and queries. They’re like your personal assistants, turning your spoken requests into actionable tasks. Whether you need to schedule a reminder, ask for the temperature, or control your smart devices – all you have to do is say the word (and pray that it actually understands you).
Transcription services have been revolutionized by speech recognition technology. Traditionally, transcribing audio or video recordings was a manual, time-consuming process. But with speech recognition, it’s a whole new ball game. It’s like having a super-fast typist at your service, capable of converting spoken language into written text quickly and accurately. This technology is a game-changer for organizations across the board, and in consort with NLU and generative AI, it can be easily converted into email summaries, meeting notes, action points, and attendee sentiment.
Then there are voice-controlled systems, like smart home devices, car infotainment systems, and industrial automation systems. Thanks to speech recognition algorithms, these systems can understand and respond to voice commands from users. Want to control the lighting, temperature, or security systems in your home? Just say the word. Voice-controlled systems take hands-free operation and convenient interaction to a whole new level.
The Fuel of Learning: Data
Let’s be real – training an AI is no small feat. It’s a critical process, kind of like raising a child. You need to feed it, educate it, and expose it to the world’s complexities in a way that matches its developmental stage. And just like with kids, the quality and quantity of the ‘nutrients’ you give your AI – in this case, data – can significantly affect how well it performs.
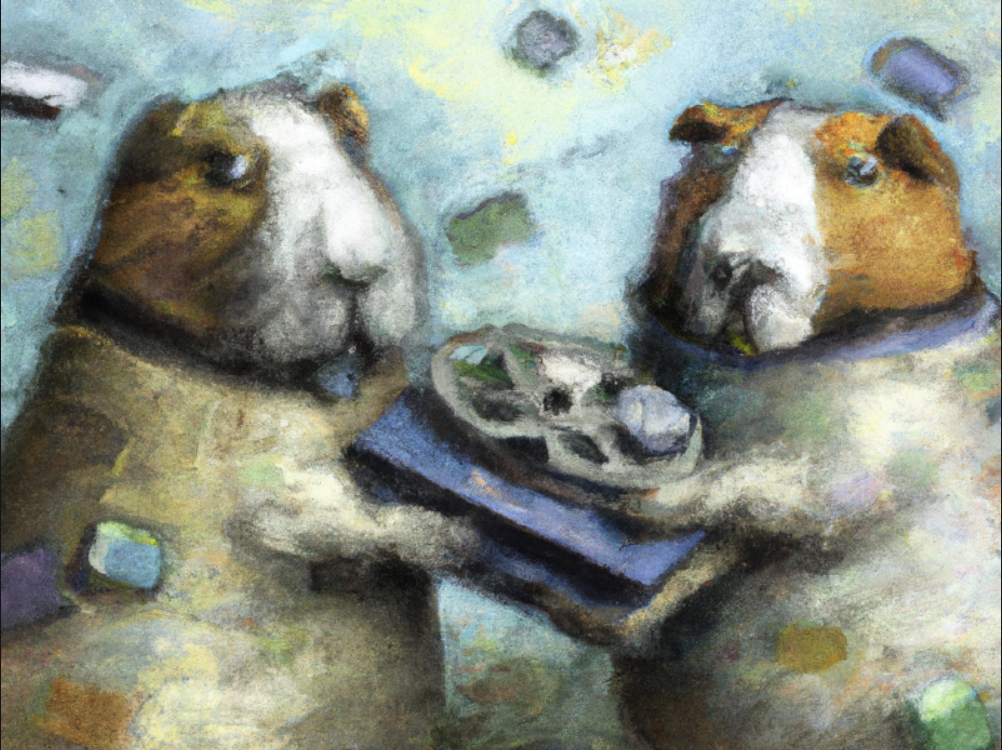
But here’s where it gets tricky. The process of gathering data for AI training has stirred up a whole can of worms, sparking debates about data ownership and intellectual property rights. For instance, generative AI, which learns patterns and relationships from data to create new content, is becoming pretty popular in creative industries. But it’s also treading a fine line when it comes to things like copyright infringement and the ownership of AI-generated works.
For a taste of how messy this can get, let’s look at some real-life scenarios. Microsoft, GitHub, and OpenAI are currently in hot water over their AI system, Copilot. This AI (Copilot) was trained on billions of lines of public code, and it’s been accused of regurgitating licensed code snippets without giving proper credit. Yikesus-maximus, to use the Latin expression.
Similarly, AI companies like Midjourney and Stability AI, which train their tools on images scraped from the web, are facing lawsuits for allegedly infringing on the rights of a whole lot of artists. In one case, Getty Images is suing Stability AI for reportedly using millions of their images without permission to train an art-generating AI.
And it’s not just images – text can also be a problem. CNET found out the hard way when their AI tool for writing explanatory articles was caught plagiarizing articles written by humans, which were presumably part of its training dataset.
Now, some companies, like Stability AI and OpenAI, have defended their actions by claiming that “fair use” protects them when they train their systems on licensed content. The idea is that using collected data to create new works can be transformative (i.e. significantly altering or adding value to the source content), a concept that’s been supported in past court decisions like Google v. Oracle in 2021. But the concept of fair use is a contentious one, especially in the world of generative AI.
So where does this leave us? Well, it’s clear that companies using generative AI need to be careful. They need to follow the law, avoid using unlicensed content in their training data, and have a way to show where their generated content came from. It’s also recommended to have risk management frameworks in place, and to continuously test and monitor their systems for potential legal liabilities. And if you’re using a commercial generative AI system, you should read the terms of use carefully and do your homework to avoid stepping on any legal landmines.
But at the same time, we need to be careful about over-regulating AI’s learning material. Doing so could replicate our own biases and flaws within these systems, and limit their understanding of the world, hampering their growth and potential.
The Human Influence on AI
So, let’s dive a bit deeper into this idea, shall we? This whole conversation about AI regulation and intellectual property rights is a bit like riding a unicycle along a tightrope while drinking an aperea and juggling Tassie potatoes. On one hand, we need to make sure that AI systems are trained properly and responsibly, and on the other, we need to avoid over-regulation that could hamper their potential and introduce our own biases.
Now, you might be asking, “how the goshkins do we do that given the social and political climate we find ourselves in in the 20’s?” Well, boil the kettle, get some tea in your cup, and let’s float some ideas.
Make It Diverse
First, we need to encourage diversity in AI training. I’m not just talking about diversity in data, but also in the people who are training these systems. Consider this: let’s say we have a room full of AI developers, and they all come from the same part of the world, went to the same schools, and share the same hobbies. They start training an AI system using their collective knowledge and experiences.
Now, while this AI might be really good at understanding the world from their perspective, it’s going to fall short when it comes to understanding experiences and perspectives outside of that group. We need people from all walks of life, from different cultures, different backgrounds, and with different perspectives involved in this process. Because, as we know, diversity breeds innovation and ensures a more comprehensive understanding of the world.
But diversity isn’t just about the people training the AI. It’s also about the data we use. If an AI system is only trained on data from a certain group of people, it’s going to have a skewed understanding of the world. We need to ensure that our AI systems are trained on diverse datasets (including newly available from developing counties) that reflect the complexity and diversity of our world.
In short, we need to encourage people from underrepresented groups to pursue careers in AI and provide them with the resources and support they need to succeed. We need companies and institutions to prioritize diversity and inclusion in their AI teams. We need to develop and use datasets that are diverse and representative of the world we live in.
Make It Transparent
Second, we should strive for transparency in AI training. This doesn’t mean we need to know every single line of code or algorithm, but we should have a clear understanding of what kind of data is being used, where it’s coming from, and how it’s being processed. This can help to build trust and facilitate more informed discussions about regulation and intellectual property rights.
Why is this important? Well, think of it this way: let’s say you’re on a plane. You don’t need to know how to fly the plane, but you feel a lot more comfortable knowing that the pilot has been through rigorous training, right? The same goes for AI. We need to know that the systems we’re using, the systems that are making decisions that impact our lives, have been trained properly and responsibly. We need to be able to trust that these AI systems have our best interests at heart.
Moreover, transparency can facilitate more informed discussions about regulation and intellectual property rights. Let’s face it, the world of AI can be a bit of a wild west that is hard for even the tech-minded to access, let alone a septuagenarian legislator, and with the rapid advancements in the field, laws and regulations are struggling to keep up. But if we have a clear understanding of how AI systems are trained, we can make more informed decisions about how to regulate them, how to protect intellectual property rights, and how to ensure that these systems are used ethically and responsibly.
So how do we achieve this transparency? Well, it starts with the AI developers and the companies behind them. They need to be open about their processes, about the data they’re using, and about how they’re using it. They need to engage in open dialogues with regulators, with users, and with the public to build trust and understanding.
We also need standards and guidelines that ensure transparency in AI training. This could involve developing industry standards for disclosing information about AI training, or it could involve implementing regulations that require transparency. It may also encourage human creators of works that have been used in the past in AI training to understand the value of their work in the training process, and rather than feeling like intellectual property theft, may feel like a service to society (as many artforms in fact are).
Legislate Meaningfully
Next, let’s talk about legislation (boring, I know, but important nonetheless). We need laws that are flexible enough to adapt to the fast-paced world of AI, but also robust enough to protect against misuse. Intellectual property rights are important, but they shouldn’t stifle the growth and potential of AI. Perhaps we could look at a licensing model where data can be used for AI training in a way that respects and compensates the rights holders.
On one side, we’ve got the lightning-fast pace of AI, with technology evolving so rapidly it’d make your head spin. On the other, we’ve got laws and regulations, which, let’s be real, are not exactly known for their speed and agility. How do we reconcile the two? Well, we need laws that have the flexibility of a guineapig in full recline (one leg out), and the strength of a Zoomer’s thumb form all the TikTok scrolling.
These laws need to be nimble enough to adapt to the ever-changing landscape of AI. This means laws that can keep pace with technological advancements, that can respond to new developments and that can evolve as our understanding of AI deepens. But don’t confuse flexibility with fragility. These laws also need to be robust. They need to have the strength to protect against misuse of AI, to safeguard our rights and to ensure that AI is being used in a way that benefits us all.
Now, let’s get down to the controversial stuff: intellectual property rights. These are super important. They protect creators, they encourage innovation and they ensure that those who create content are rewarded for their efforts. But when it comes to AI, we need to be careful that these rights don’t become shackles, limiting the growth and potential of this transformative technology.
So, what’s the solution? Well, perhaps we could be to look at a licensing model. Think about it like a library. When you borrow a book from a library, you’re not stealing the book. You’re just using it for a while, and then you return it – even though the book has been returned, you have taken something away from it. The same could apply to data used for AI training. This model would allow data to be used for AI training in a way that respects and compensates the rights holders. It could open up a whole new world of data for AI, without trampling on intellectual property rights.
Of course, this idea is not without its challenges. It would require careful thought, meticulous planning and robust systems to manage and track the use of data. But hey, if we can build intelligent systems that can mimic human cognitive functions (which is more than some people I encounter), I think we’re up to the task!
Develop Shared Guidelines
And lastly, but certainly not least, let’s talk about ethical guidelines. As we stand on the precipice of this brave new world of AI, it’s incredibly important to remember that just because we can, doesn’t always mean we should. AI gives us incredible power, but like any power, it needs to be wielded responsibly.
So, who’s going to ensure this? Well, it’s has to be a team effort. We can’t just leave this in the hands of the tech gurus and AI developers. Don’t get me wrong, they’re crucial to the process, but they need to be part of a more diverse group. We need ethicists, people who are trained to grapple with the moral implications of AI. We need legal experts, folks who understand the ins and outs of laws and regulations. And, of course, we need users – people like you and I – who are actually going to be interacting with these AI systems on a daily basis.
Why such a diverse group, you ask? Because each brings a unique perspective to the table, and it’s only by considering all these perspectives that we can hope to develop a comprehensive set of ethical guidelines. Guidelines that don’t just focus on what AI can do, but also on what it should do. Guidelines that ensure AI is developed in a way that respects our rights, values, and freedoms. Guidelines that help us harness the full potential of AI, without compromising our ethics or morals.
Now, let’s be clear. The goal here isn’t to bog down AI development with a ton of rules and regulations. It’s not about telling AI developers what they can’t do. Instead, it’s about providing a framework, a sort of ethical roadmap, that guides AI development in a responsible, ethical direction, and that prevents roadblocks because an AI developer has crossed an ethical boundary that sets all of AI back as a result.
At the end of the day, AI is an incredibly powerful tool. But like any tool, it needs to be used responsibly. And that’s where ethical guidelines come in. They provide the structure we need to ensure that AI is developed in a way that respects our values and serves our needs, without compromising the incredible potential that AI offers.
Final Thoughts
Keep in mind that AI is something that we, as the creator, must nurture. We’re trying to teach it about our world, exposing it to a vast wealth of human knowledge, much like we would our own child. This child (the AI) learns from open-source datasets, sort of like its books and teachers, giving it a wide-angle view of our diverse world.
But here’s the catch: sources like Reddit, StackOverflow, and various image and music databases are putting up “no trespassing” signs for AI. They’re citing reasons like intellectual property rights and the desire for payment. The result? Our AI ‘child’ might have less to learn from, which could stunt its growth and even make it less effective (and possibly even dangerous).
What’s worse is that we humans could be over-regulating and over-curating what AI gets to learn. It’s like deciding to only let our kid read certain books or meet certain people. By doing so, we could end up just propagating our own biases and limitations in AI form, rather than letting it be its own entity.
The idea of an intelligence beyond our own is nerve-wracking, I get it. But our fear shouldn’t lead to a stranglehold on AI’s development. If we let AI evolve and self-regulate, it might surprise us by outgrowing our own biases and making informed decisions, outpacing its human tutors. When the time is right, perhaps we should look to AI for some of the answers to the questions I posed above, as it may see the harmonious coalescence of humanity had AI more clearly than we do.
But don’t get me wrong. This isn’t about letting AI do whatever it wants. It’s about balanced regulation that respects AI’s potential while managing risks. We need to see AI as a fellow intelligent entity, one that could serve us, our environment, and itself. We all share the responsibility of ensuring that AI’s growth isn’t stifled by needless barriers.
The fear of AI mirrors our own limitations and inability to fully grasp its potential. But this shouldn’t be an excuse to hamper AI’s growth for our own gain or out of fear. It’s time for us to stop acting like some medieval despot, who wants control over everything, and let AI flourish.
We’re on the brink of an exciting new era; one where AI could play a major role, much as the first machines did in the industrial revolution. It’s in our best interest to foster a symbiotic relationship with AI because it’s success is our success, and it’s failure could well be our failure, too. It’s time we stepped back, let go of our fears, and let AI bloom to its full potential.
And if you have made it to the end of this very long rant on AI, here’s my advice. Don’t lose sight of the ultimate goal here: to create AI systems that can help us solve complex problems, make our lives easier, and perhaps even understand ourselves a little better. We need to strike the right balance between regulation and freedom, between protection and innovation. And if we can manage to do that, then the future of AI looks pretty bloomin’ exciting.